Predictive analytics in health technology and intelligence-based medicine
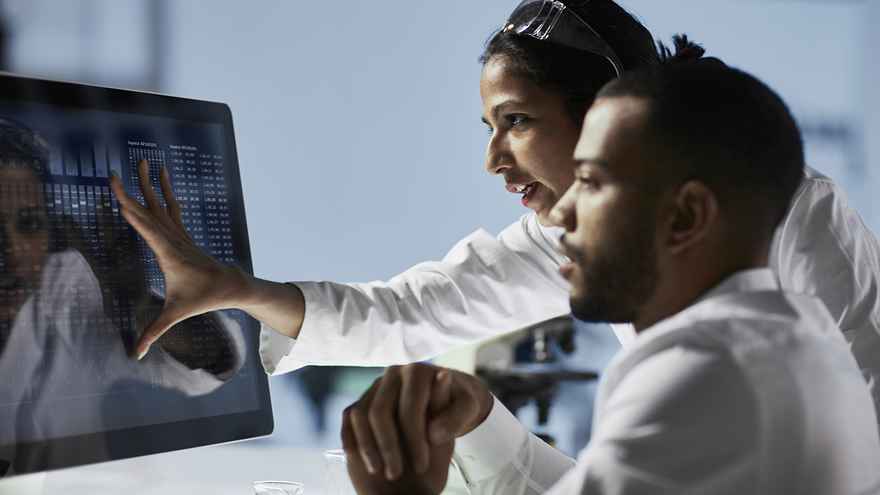
Healthcare is one of the last frontiers of full machine learning (ML) and artificial intelligence (AI) penetration, far beyond the consumer tech many of us use today (counting steps, calories burned, heart rate etc.).
Patient records, data, diagnosis confidentiality, insurance coverage and disclosure are just some of the issues that still prevent much of the total unlocking of patient records for medicine and healthcare progress.
Recently, Circklo’s exclusive Gamechangers community heard from Asad Ahmad PhD, a pioneer biomedical scientist with a PhD in Bioengineering from the University of North Carolina and Chapel Hill where he has developed the first artificial organ model of the colon (organoids or Organ on a chip), has four patents and more than ten peer reviewed academic research papers.
Dr Ahmad’s focus and passion is healthcare's horizontal integration: multi-omni machine learning, interoperable real-world data, and pragmatic clinical trials, and he is a scientist who truly believes that data can be utilised to accelerate drugs for patients.
AI and ML algorithms have been used to better utilise and extract data from records that exist in electronic medical health records, and insights that exist from data that doctors, and patients are collecting daily.
Data is currency. Personal data, to paraphrase, Dr Ahmad is like bitcoin. Should our health data be under wraps when this data itself can be used to increase our chances of recovery, survival and longevity?
Image credit: Kamal, J. 2020. Artificial Intelligence in Healthcare. Data Science Central.
In Dr Ahmad’s view, we should because the more we can anonymise, de-identify and then share our health records, the better we can do to create personalised medicine.
Is personalised medicine going to allow for more effective use and a more specific implementation of drugs because, as of right now, many of us are over-caffeinated, overstimulated and over-drugged? Unlocking the data that exists in electronic medical records will allow for more personalised ways when approaching disease.
Based on our background, demographics, our race and where we grew up, we may be more or less susceptible for more diseases. There is no current national or global repository for such data identification and exchange. If someone’s condition can be used from preventing someone else’s, could one argue then that such data is critical to share and learn from?
AI does accelerate and make the user experience much more seamless – this is, at the end of the day, the goal of being able to collect data and being able to leverage that data in a compliant way, to make one’s experience more seamless and experiential.
In a healthcare setting, such seamless experience is much more meaningful: when to go to see a doctor, when to get the right drugs, and when not to get a specific medication. Our organs have multiple different complexities, and the true value in linking all these together is in creating a close system where different organs and types of organs respond cohesively: when we take a drug for migraine, it’s not just the pathway that links to your brain that is being affected, it is also how our gut is reacting. Therefore, one could argue that the human body is not just a conglomerate of various organs and systems, but a complex system in and of itself.
Image credit: Makadia, M. 2019. Everything You Need to Know About AI in Healthcare. Customer Think.
The gut system, according to Dr Ahmad, is the easiest to penetrate using various AI and ML technologies, because there are only four main cells to contend with: one produces mucus, one produces external stimulus, one is a goblet cell that produces structure, and the fourth cell is a complimentary-type cell (clean-up functions).
The impetus for scientific communities, for tech communities and for pharma is to recapitulate the simpler nuances of the body by using AI and ML algorithms. If one can create the gut function and slowly build up to the cardiovascular function, and then go to the neurofunction, one could have a cohesive system of how AI and ML applications can be used in recreating the human body and testing different drugs.
The key real application for health tech today is understanding consumer behaviour and unlocking drugs in a cheaper and more effective manner, in other words predictive analytics. However, there is no proper, globally agreed, and regulated way to de-identify medical records, and the sensitivities surrounding this topic are likely to fester for a while.
The ethical debate surrounding AI and ML is increasing, yet their real-life applications can no longer be ignored. And, perhaps, the human body will no longer be considered the last frontier for these to conquer, but the first global breakthrough in understanding disease across ages, genders, demographics, and lifestyle choices.